Premium Only Content
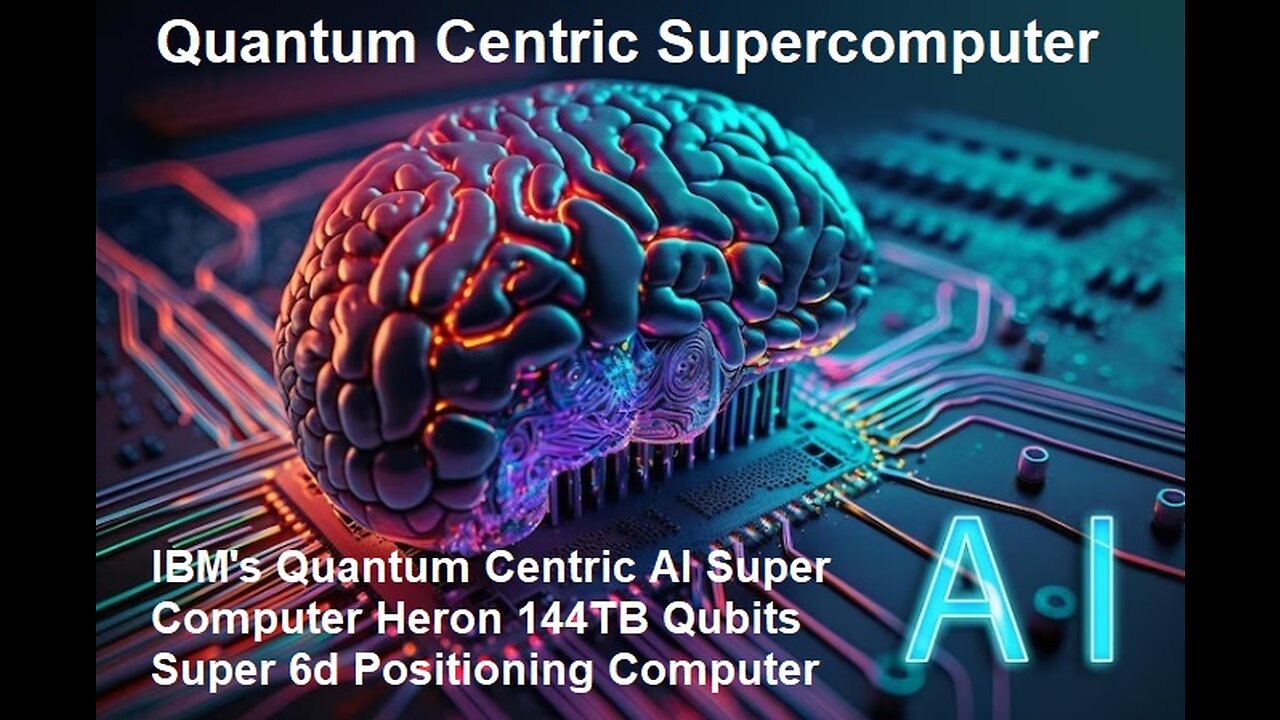
IBM's Quantum Centric Supercomputer Heron 144TB Qubits Superposition Computer
Welcome Now New AI Quantum Centric Supercomputer Heron 144TB and its is running now and where we are explore now the fascinating world of science and technology now and the 4d universe! From the inner workings of the human body 5d to the outer reaches of 6d space and time we delivery into the latest and most interesting discoveries that are shaping our 8d world now. Whether you're a science buff or just looking for some mind-blowing facts, we've got you covered. Join us in this video as we uncover the mysteries of the world around us and discover new frontiers in the fields of science and technology. Get ready for a journey that's both educational and entertaining.
For $100 million it better beat an Nvidia A100 IBM plans spend $100 million to build a 100,000 qubit "quantum-centric supercomputer" allegedly capable of solving the world's most intractable problems by 2033 and it's tapped the Universities of Tokyo and Chicago for help and the real number now is more like 6 billion dollars in today money 2023.
Quantum computing today is a bit of a catch-22. The jury is still out as to whether the tech will ever amount to anything more than a curiosity – but if it does, nobody wants to be the last to figure it out. And IBM – which already plans to invest $20 billion into its Poughkeepsie, New York, campus to accelerate the development of, among other things, quantum computers – clearly doesn't want to be left behind.
If IBM is to be believed, its quantum supercomputer will be the foundation on which problems too complex for today's supercomputers might be solved. In a promotional video published Sunday, Big Blue claimed the machine might unlock novel materials, help develop more effective fertilizers, or discover better ways to sequester carbon from the atmosphere. You may have heard this before about Watson.
But before IBM can do any of that, it actually has to build a machine capable of wrangling 100,000 qubits – and then find a way to get the system to do something useful. This is by no means an easy prospect. Even if it can be done, the latest research suggests that 100,000 qubits may not be enough – more on that later.
IBM solicits help for quantum quest
To put things in perspective, IBM's most powerful quantum system to date is called Osprey. It came online late last year and featured a whopping 433 qubits. At least as it's imagined today, the quantum part of IBM's quantum supercomputer will be made up of four 25,000 qubit clusters.
This means to achieve the stated 2033 timetable, IBM's quantum systems will need to increase the number of usable qubits by roughly 50 percent every year for the next decade, and then build and connect them using both quantum and classical networks.
The situation may actually be worse. It appears that the system will be based on Big Blue's upcoming 133 qubit Heron system which, while smaller, employs a two-qubit gate architecture IBM claims offers greater performance.
To help IBM reach its 100,000 qubit goal, the biz has solicited the Universities of Tokyo and Chicago for help. The University of Tokyo will lead efforts to "identify, scale, and run end-to-end demonstrations of quantum algorithms." The university will also tackle supply chain and materials development for large-scale quantum systems, like cryogenics and control electronics.
Meanwhile, researchers at the University of Chicago will lead the effort to develop quantum-classical networks and apply them to hybrid-quantum computational environments. As we understand it, this will also involve the development of quantum middleware to allow workloads to be distributed across both classical and quantum compute resources.
To be clear, IBM's 100,000 qubit target is based entirely on its roadmap and the rate at which its boffins believe they can scale the system while also avoiding insurmountable roadblocks.
"We think that together with the University of Chicago and the University of Tokyo, 100,000 connected qubits is an achievable goal by 2033," the lumbering giant of big-iron computing declared in a blog post Sunday.
What good is a 100,000 qubit quantum computer?
Even if IBM and friends can pull it off and build their "quantum-centric" supercomputer, that doesn't necessarily mean it will be all that super. Building a quantum system is one thing – developing the necessary algorithms to take advantage of it is another entirely. In fact, numerous cloud providers, including Microsoft and OVH Cloud have taken steps to help develop quantum algorithms and hybrid-quantum applications in preparation for when such utility-scale systems become available.
And according to a paper penned by researchers from Microsoft and the Scalable Parallel Computing Laboratory, there's reason to believe Big Blue's 100,000 qubit quantum computer might not be that useful.
The researchers compared a theoretical quantum system with 10,000 error correcting qubits – or about a million physical qubits – to a classical computer equipped with a solitary Nvidia A100 GPU. The comparison revealed that for the quantum system to make sense, the algorithms involved need to achieve a greater-than-quadratic speed up.
Assuming IBM is talking about 100,000 physical qubits – it's not specified — that'd make its machine about one tenth the size of the theoretical system described in the research paper. We've reached out to IBM for clarification and we'll let you know if we hear anything back.
That said, there are some workloads that show promise – as long as the I/O bottlenecks can be overcome. While the researchers found that drug design, protein folding, and weather prediction are probably out of the question, chemical and material sciences could benefit from an adequately large quantum system. So, IBM's pitch of using a quantum supercomputer to develop lower-cost fertilizers might not be the craziest idea ever.
Vodafone worries about sci-fi tech's potential to break encryption British-based bank HSBC is to test a pilot quantum-secured metro network in London, in the hopes of preparing for potential security threats in the future. Meanwhile, Vodafone is looking to protect users of its phone network against a potential quantum threat to encryption codes.
HSBC said it intends to use a quantum-secured metro network to evaluate secure transmission of data across standard fiber-optic cables between its global headquarters at Canary Wharf in London's Docklands, and a datacenter 62km (38 miles) away in the county of Berkshire.
According to the bank, it will make use of the network for carrying financial transactions and secure video communications, as well as for one-time-pad encryption, and will be testing the network's use in edge computing by connecting an AWS Snowball Edge device.
The network in question was announced last year by BT and uses quantum key distribution (QKD) over standard 10Gbps fiber-optic connections to secure end-to-end transmissions. The QKD hardware and the key management systems are provided by Toshiba, and the whole system is a three-year commercial trial to gauge the viability of a quantum-secured metro network.
BT has already had accounting firm EY testing out the network to link two of its sites in London, one at Canary Wharf and one other near London Bridge.
QKD is a method of securely distributing encryption keys by encoding the information into the quantum states of individual photons. Once this is accomplished, information can be exchanged normally using the keys to encrypt transmissions. The tricky part is building the network so the QKD can use the same optical fibers as the data traffic rather than needing dedicated lines.
HSBC said its exploration of quantum-secure communications is intended to glean crucial evidence into the effectiveness of the technology and drive the development of future applications for financial security.
"Our customers, clients and employees expect us to have safe and secure operations and resilient cybersecurity, so we must stay ahead of the curve," Colin Bell, CEO of HSBC Bank and HSBC Europe, said in a statement.
HSBC is recruiting highly trained experts, while running the trials, and investing in strategic partnerships to explore how to deploy these technologies as they develop, he added.
The bank said it processed 4.5 billion payments for customers last year, worth an estimated £3.5 trillion, and those transactions rely on encryption to protect customers from potential fraud or theft.
Quantum-safe VPN, anyone?
Meanwhile, Vodafone is more worried about the potential of quantum systems to be able to break encryption codes and is testing out a quantum-safe virtual private network (VPN) for smartphones.
The telecom giant said it has joined forces with SandboxAQ, a startup spun off from Google's parent company Alphabet last year, to carry out proof-of-concept testing for a quantum-safe VPN using standard smartphones.
Vodafone said the VPN had been adapted to use encryption codes developed by the US National Institute of Standards and Technology (NIST), known as post-quantum cryptography (PQC) algorithms, which are supposed to be resistant to cracking by quantum methods.
We trust that Vodafone and Sandbox AQ are not using the NIST algorithm that was cracked in an hour by security researchers last year.
According to Vodafone's Head of Research and Development, Luke Ibbetson, the company is worried about so-called "Store Now, Decrypt Later" attacks, where malicious actors may harvest encrypted sensitive communications hoping to decode it using quantum systems in the future.
"Although cryptographically relevant quantum computers may remain some years off, the threat posed by quantum-empowered attackers is already here today," Ibbetson claimed.
Vodafone said it has been testing multiple PQC algorithms with an eye to how they may impact performance during activities such as web browsing, chat app use, video and audio streaming, and mobile gaming. It reports that a hybrid cryptographic approach using classical and "best-fit" PQC algorithms had minimal impact on the quality of service, while still providing post-quantum security.
The telco acknowledged that a transition to PQC will take time and resources, but said it is important to start acting now. Vodafone said it will continue to test new solutions and combine its work with broader industry groups to address the need for global standards to protect data.
You can’t steal what you can’t access and we hope ? Microsoft has vowed to bulk up security around its Azure DevOps cloud services developers use to build their applications and manage their software projects.
The security enhancements are part of the larger roadmap for Azure DevOps that the cloud giant laid out this week that also includes additions to Azure Boards – for tracking ideas throughout the development lifecycle – and Azure Pipelines to automatically build and test code.
The changes also come as Microsoft bolsters its Entra suite of cloud-based identity and access services, not only by ditching the Azure AD name in favor of Entra ID – a move not fully embraced by all users – but also through its first offerings in the fast-growing security services edge (SSE) space.
One focus for Redmond is the GitHub code repository, which like other code bases – such as NPM and the Python Package Index (PyPI) – has become a target for criminals in supply chain attacks aimed at getting developers to inadvertently dropping malicious code into their applications.
GitHub Advanced Security (GHAS) for Azure DevOps is a suite of tools developers can use to protect their Azure Repos repositories and Pipelines. These include secret scanning to detect such secrets as credentials already in Azure Repos and ways to keep developers from accidentally pushing new secrets and dependency scanning, so they can find known vulnerable open-source packages and fix any problems.
Also in GHAS – which is in public preview and integrated into Azure DevOps – is code scanning, which uses GitHub's CodeQL semantic analysis engine to identity app security flaws in the source code.
Authentication on the menu
Identity and authentication also will factor heavily in what Microsoft does through at least the rest of the year. The vendor for several years has banged the drum for improved authentication tools – such as ModernAuth and passkeys – as identity becomes a key focus for cyber-attackers.
In Azure DevOps, a key risk is credential theft.
"Azure DevOps supports many different authentication mechanisms, including basic authentication, personal access tokens (PATs), SSH, and Azure Active Directory access tokens," the company wrote. "These mechanisms are not created equal from a security perspective, especially when it comes to the potential for credential theft."
Criminals can use leaked credentials like PATs to get into organizations using Azure DevOps and access source code, launch supply chain attacks, or compromise the infrastructure.
Microsoft will also release Workload Identity federation for Azure Deployments, first in public preview in the third quarter and then generally by the end of the year. Developers are wary of storing secrets like passwords or certificate in Azure DevOps because they become vulnerable to theft when service connections in Azure DevOps are updated.
Protection through federation
Azure will use the Open ID Connect protocol to support workload identity federation and create service connections in Azure Pipelines that don't access secrets and which are backed by managed identities with federated credentials in Azure AD.
"As part of its execution, a pipeline can exchange its own internal token with an AAD token, thereby gaining access to Azure resources," Microsoft wrote. "Once implemented, this mechanism will be recommended in the product over other types of Azure service connections that exist today."
Microsoft also will support granular scopes to limit the operations of Azure AD OAuth applications, such as viewing source code or configuring pipelines, when connecting to Azure DevOps.
Also by the end of 2023, Microsoft will let applications use managed identities and service principals when integrating with Azure DevOps through REST APIs and client libraries. Most applications now integrate through PATs.
"This highly requested feature offers Azure DevOps customers a more secure alternative to PATs," Redmond wrote. "And Managed Identities offer the ability for applications running on Azure resources to obtain Azure AD tokens without needing to manage any credentials at all."
Microsoft takes to SSE
All this comes the same week Microsoft made changes in its Entra suite. The first, as we've documented, was the name change from Azure AD to Entra. Another key one was the rollout into public preview of Entra Internet Access and Entra Private Access, Redmond's first SSE offerings.
Secure Access Service Edge (SASE) hit the scene several years ago when enterprises, faced with having to manage security and identity wirelessly, wanted vendors to converge software-defined WAN and network security functions, such as zero trust, firewall-as-a-service (FWaaS), and cloud access security broker (CASB), into a cloud service.
SSE emerged during the pandemic, essentially ditching the SD-WAN functions and unifying CASB, zero trust, and secure web gateway (SWG) into a service. Microsoft is coming into this space late, with vendors like Cisco, Zscaler, and Palo Alto Networks, among others, already a year or two ahead.
However, Microsoft's sheer gravitational pull will help it gain market share, as shown by the drop in share prices of Cloudflare, Palo Alto, and Zscaler right after Microsoft announced its SSE move.
The US government on Tuesday added commercial spyware makers Intellexa and Cytrox to its Entity List, saying the duo are a possible threat to national security.
According to the Feds, Greece's Intellexa SA, Ireland's Intellexa Limited, North Macedonia's Cytrox AD, and Hungary's Cytrox Holdings are allied companies that developed and sold software that could be used by clients to infect and monitor other people's electronic devices and equipment. This "is acting contrary to the national security or foreign policy interests of the United States," as the US Dept of Commerce put it [PDF].
Adding Intellexa and Cytrox to the Entity List places export restrictions on the software vendors as part of the Biden administration's ongoing crackdown against commercial surveillance technology. It is now impossible for US organizations to do business legally with those placed on the list without special permission from Uncle Sam; the list effectively cuts off Intellexa et al from America.
The move also follows warnings from cybersecurity researchers about abuses committed using the firms' snooping products.
Alliances
Google's Threat Analysis Group (TAG), Cisco Talos, and Canadian nonprofit Citizen Lab have published reports on Cytrox's Predator and Alien spyware, which we're told have been used by the biz's customers to target politicians, journalists and activists.
Like similar snoopware package Pegasus, whose maker NSO Group was added to the federal Entity List in 2021, Predator and Alien have been documented exploiting zero-day flaws and other vulnerabilities to infect and take over Android phones and Apple iOS devices to spy on users and extracting data.
According to Citizen Lab, Cytrox is part of Intellexa, which formed the "Star Alliance of spyware" in 2019 to compete against NSO. Although, as the nonprofit noted in a 2021 report, "the specific link between Cytrox and Intellexa, as well as other companies in the 'alliance,' remains murky at best."
Last year, Google TAG said Cytrox sold zero-day exploits to government-backed snoops who used them to deploy Predator in at least three campaigns in 2021. The TAG team believes the buyers of these exploits are in Egypt, Armenia, Greece, Madagascar, Côte d'Ivoire, Serbia, Spain, Indonesia, and possibly other countries.
"We assess with high confidence that these exploits were packaged by a single commercial surveillance company, Cytrox, and sold to different government-backed actors who used them in at least the three campaigns," Google security researchers Clement Lecigne and Christian Resell said.
And in March, Meta's former security policy manager, who split her time between the US and Greece, sued the Hellenic national intelligence service for compromising her phone and deploying Predator spyware. The case is as yet unresolved.
"This rule reaffirms the protection of human rights worldwide as a fundamental US. foreign policy interest," Deputy Secretary of Commerce Don Graves said in a statement today. "The Entity List remains a powerful tool in our arsenal to prevent bad actors around the world from using American technology to reach their nefarious goals."
Google, Citizen Lab, and other digital privacy advocates have called on Congress to weigh in on spyware, asking for sanctions and increased enforcement against surveillanceware makers.
The Commerce Department updated its list a few months after US President Joe Biden issued an executive order to (somewhat) prohibit the US government from using commercial spyware. Meanwhile, the Feds continue to promote the sale of American-approved commercial spyware to foreign governments at the expense of US taxpayers.
When they're done, there might be a commercially viable system sometime after 2030 and maybe ? Intel is providing its latest quantum chips to research facilities, including some US universities, in order to drive the development of technology for quantum computers including techniques for handling multiple qubits.
The Santa Clara chipmaker is delivering the Tunnel Falls 12-qubit test chip to research laboratories for experimentation. Among the first to get access will be the the University of Maryland, the University of Rochester, and the University of Wisconsin at Madison and on the national lab front, Sandia.
"What we want to do here is build a research ecosystem," Intel's director of Quantum Hardware, James Clarke told us.
"If we take a look at all the big advances we've seen in the semiconductor community, both from a performance perspective of transistors, but also an ecosystem of maybe the tools to build the transistors, they've all gone through this space, where we need an ecosystem of people studying these devices to accelerate quantum to hit that milestone in the next decade."
The milestone Clarke is referring to is a commercially relevant quantum system, which he doesn't believe will happen "until well after 2030," but Intel is laying the groundwork now for the technologies to try to make it happen.
Tunnel Falls is a 12-qubit test chip based on Intel's silicon quantum dot technology. It is manufactured at Intel's D-1 R&D fabrication plant in Oregon as part of a standard 300mm wafer using similar processes to the company's normal chips.
This is part of Intel's approach to developing quantum technology, which is to "ride the coattails" of the semiconductor industry's decades of painstaking work to miniaturize and perfect CMOS transistors, Clarke explained.
"We have focused our quantum technology on the baseline technology of our CMOS processes, we're building our quantum chip using all the tools that we have to make our transistors," he said.
Although it sounds esoteric, the silicon quantum dot technology is actually just based on single electron transistors. A single electron gets trapped under the transistor gate, and that electron has a quantum property called spin that is either up or down, which is used to represent the zero or one of a qubit.
There are also sensor devices on the chip near the individual gates, which indicate whether the electron is in the spin up or spin down state.
As a test chip, Tunnel Falls implements a variety of different structures, representing different skews on the qubit design. All of this has to work in a refrigerator chilled to 1.6 Kelvin (-271 Celsius or -456 Fahrenheit).
Clarke claims Intel has seen a 95 percent yield rate across the wafer, plus "a voltage uniformity in how these devices turn on at a very low temperature that's actually quite similar to a CMOS logic process." And while the quantum performance is still being characterized, "the yield and uniformity is consistent with that of an advanced [CMOS] process," he claimed.
The next step now is to collaborate with the research laboratories to build an ecosystem that can work towards realizing workable quantum systems.
"Tunnel Falls is based on CMOS technology. That's how we're going to move fast. That's how we're going to turn the 42 year timeline for transistors into a much shorter timeline for qubits just piggybacking on what we know with transistors, and that sets us apart from others," Clarke said.
"This chip will enable novel experiments and the creation of new techniques for working with multiple qubits. And while we are testing this chip, just like with any other Intel process, we're taping out our next design, and we're designing the next chip beyond that.
"We will continue to improve the performance of Tunnel Falls. It happens almost weekly. And then I would say as a teaser, we expect the next generation chip to be released in 2024."
Long term, Intel hopes to be able to deliver a complete quantum system, not just the quantum chips.
"Intel will deliver the full stack," Clarke said. "A large scale quantum computer is going to have a quantum chip plus a host of control chips, and they're going to be based on Intel technologies, it's probably going to have an HPC system or supercomputer connected to the quantum computer to process the exponential amount of data.
"And so Intel is uniquely situated here with our capability, both from an architecture and fabrication, to build all the components. But do we sell systems? Or do we offer quantum-as-a-service in the cloud? I think that's too early to tell. Let's get the quantum system first."
He warned that this is still a long way off, contrasting Intel's step-by-step approach against some rival quantum companies that have tried to go more or less directly to attempting a functional quantum system.
"I was often asked how long would it take to have a quantum computer, I said 10 to 15 years," Clarke said. "Other quantum companies were saying they'd have a commercially relevant quantum computer in five years.
"Today you can find systems of up to a couple of hundred qubits. The purpose of these systems is to begin to explore contrived applications where the power could potentially exceed that of a supercomputer.
"In several years' time, perhaps four to six years, we will be in the range of having thousands of qubits and being able to perform error correction, taking thousands of physical qubits, and running an algorithm to allow us to have a logical or long-lived qubit with which we can do substantial manipulation without losing information."
But it will likely require error correction and millions of qubits to do something commercially relevant, he insisted, and that is unlikely to happen until sometime well beyond 2030.
In-Depth Guide to Future of AI in 2023, According to Top Experts Investment and interest in AI is expected to increase in the long run since major AI use cases (e.g. autonomous driving, AI-powered medical diagnosis) that will unlock significant economic value are within reach. These use cases are likely to materialize since improvements are expected in the 3 building blocks of AI: availability of more data, better algorithms and computing.
Short term changes are hard to predict and we could experience another AI winter however, it would likely be short-lived. Feel free to jump to different sections to see the latest answers to your questions about the future of AI.
Interest in AI has been increasing
There has been a 14x increase in the number of active AI startups since 2000. Thanks to recent advances in deep-learning, AI is already powering search engines, online translators, virtual assistants, and numerous marketing and sales decisions.
There are high value AI use cases that require further research
Autonomous driving is one popular use case with an increasing trend. As Tesla and Audi manufacture semi-autonomous vehicles today they still require drivers to control. This technology rapidly continues to improve to reach a fully automated driving level. Though Elon Musk stated “Next year for sure, we will have over a million robotaxis on the road,” in October 2019, we still don’t see robotaxis. This is because Elon Musk is the master of hype and self-driving cars have complex regulatory issues such as liability accident. Elon Musk also highlighted this issue via a tweet reply in April 2020.
However, McKinsey predicts that roughly 15% of vehicles sold in 2030 will be fully autonomous.
Automated content generation also arouse the interest of businesses and AI experts, as GPT-3 is released by OpenAI in June 2020. Compare to GPT-2, OpenAI increased the number of parameters to 175 billion from 1.5 billion. Yet, it appears GPT-3 has also weaknesses in some tasks that require a comparison between two sentences and its accuracy is less than 70% with few-shot learning. In near future, we will encounter with higher accuracy content automation solution as Natural Language Generation (NLG) technology advances.
Another use case is the conversational AI/chatbots. We commonly encounter with AI agents in customer services and call centers. However, capabilities of these agents are currently quite limited. As AI research progresses, conversational agents will improve to handle almost all customers’ tasks in the future.
AI research effort continues to grow
Between 1996 and 2016, the number of published papers on AI has increased eight times, outpacing the growth in computer science papers.
In the late 90’s AI papers accounted for less than 1% of articles and around 3% of conference publications. By 2018, the percentage of published AI papers in total papers has increased 3 times in 20 years, accounting for 3% of peer-reviewed journal publications and 9% of published conference papers.
Research may need to continue in new directions beyond deep learning for breakthrough AI research. There are AI researchers like Gary Marcus who believe that deep learning has reached its potential and that other AI approaches are required for new breakthrough. Gray outlined his observations on the limitations of AI in this paper, answered most critical arguments against his paper and put a timeline on these predictions. He expects VC enthusiasm in AI to be tempered in 2021 but expects the next AI paradigm unlocking commercial opportunities (e.g. the new deep learning) to be available some time between 2023-2027.
What are the key trends that shape the future of AI?
AI systems so far relied on these for improvement: increased computing power, availability of more data, better algorithms and better tools. In all 4 areas, there is potential for dramatic improvements though it is hard to put these against a timeline. In addition, thanks to cryptography and blockchain, it is becoming easier to use wisdom of the crowd to build AI solutions which will also facilitate AI model building.
Advances in computing power
Deep learning relies on computing power to solve more complex problems. With current technology, learning may take too long time to be beneficial. Therefore, there is need for advances in computing power. With new computing technologies, companies can have AI models that can learn to solve more complex problems.
AI-enabled chips
Even the most advanced CPU may not improve the efficiency of an AI model by itself. To use AI in cases like computer vision, natural language processing, or speech recognition, companies need high-performance CPUs. AI-enabled chips become a solution to this challenge. These chips make CPUs “intelligent” for optimizing their tasks. As a result, CPUs can work for their duties individually and improve their efficiency. New AI technologies will require these chips to solve complicated tasks and perform them faster.
Companies like Facebook, Amazon, and Google are increasing their investments in AI-enabled chips. Below you can find a chart of global equity funding for AI-enabled chip startups.
These chips will assist next-generation databases for faster query processing and predictive analytics. Industries like healthcare and automobile heavily rely on these chips for delivering intelligence. We have prepared a comprehensive, sortable list of companies working on AI chips.
Advances in GPUs
GPUs are one of the most commercially used type of AI enabled chips.
Rendering an image requires simple computing power but needs to be done on a large scale very quickly. GPUs are the best option for such cases because they can process thousands of simple tasks simultaneously. As new technologies in GPU renders better-quality images since they do these simple tasks a lot faster.
Modern GPUs have become powerful enough to be used for tasks beyond image rendering, such as cryptocurrency mining or machine learning. While CPUs usually used to do these tasks, data scientists discovered that these are repetitive parallel tasks. Thus, GPUs are widely used in AI models for efficient learning.
Quantum computing
Traditional computer systems work with binary states; 0 and 1. However, quantum computing takes this to another level and works with quantum mechanics. This enables quantum systems to work with qubits, instead of bits. While bits consist of 0 and 1, qubits consist of 0, 1 and an additional state, which includes both at the same time. This additional state enables quantum computing to be open to new possibilities and provide faster computation for certain tasks. These tasks include neural network optimizations and digital approximations.
IBM states that it will be possible to build a quantum computer with 50-100 qubits in the next 10 years. When we consider that the 50-qubit quantum computer works faster than today’s best 500 supercomputers, there is significant potential for quantum computing to provide additional computing power.
Advances in data availability
This is a point that does not need to be explained in much detail. Data availability has been growing exponentially and is expected to continue to do so with increasing ubiquity of IoT devices.
Advances in algorithm design
While the capabilities of AI improve rapidly, the algorithms behind AI models will also evolve. The advancements in the algorithm designs will enable AI to work more efficiently and be available to more people with less amount of technical knowledge. Below you can find the prominent advancements in AI algorithm designs.
Explainable AI (XAI)
One of the main weak points of AI models is its complexity. Building and understanding an AI model requires a certain level of programming skills and, it costs time to digest the workflow of the model. As a result, companies usually benefit from the results of AI models without understanding their workflow.
To solve this challenge, Explainable AI makes these models understandable by anyone. XAI has three main goals:
How the AI model affects developers and users
How it affects data sources and results
How inputs lead output
As an example, AI models will be able to diagnose diseases in the future. However, doctors also need to know how AI comes up with the diagnosis. With XAI, they can understand how AI makes its analysis and explain the situation to their patients accordingly. If you are interested, you can read more about XAI from our in-depth guide.
Transfer learning
Transfer learning is a machine learning method that enables users to benefit from a previously used AI model for a different task. In several cases, it is clever to use this technique for the following reasons:
Some AI models aren’t easy to train and can take weeks to work properly. When another task comes up, developers can choose to adopt this trained model, instead of creating a new one. This will save time for model training.
There might not be enough data in some cases. Instead of working with a small amount of data, companies can use previously trained models for more accurate results.
As an example, an AI model that is well-trained to recognize different cars can also be used for trucks. Instead of starting from scratch, the insight gained from cars will be beneficial for trucks.
Reinforcement learning (RL)
Reinforcement learning is a subset of machine learning which aims AI agent to take action for maximizing its reward. Rather than traditional learning, RL doesn’t look for patterns to make predictions. It makes sequential decisions to maximize its reward and it learns by experience.
Today, the most common example of RL is Google’s DeepMind AlphaGo which has defeated the world’s number one Go player Ke Jie in two consecutive games. In the future, RL will also be available in fully automated factories and self-driving cars.
Self-Supervised Learning (Self-Supervision)
Self-supervised learning (or self-supervision) is a form of autonomous supervised learning. Unlike supervised learning, this technique doesn’t require humans to label data, and it handles the labeling task by itself. According to Yann LeCun, Facebook VP and chief AI scientist, self-supervised learning will play a critical role in understanding human-level intelligence.
While this method is mostly used in computer vision and NLP tasks like image colorization or language translation today, it is expected to be used more widely in our daily lives. Some future use cases of self-supervised learning include:
Healthcare: This technique can be used in robotic surgeries and estimating the dense depth in monocular endoscopy.
Autonomous driving: It can determine the roughness of the terrain in off-roading and depth completion while driving.
Advances in AI building tools
Though these are not novel algorithms, they can reduce the time to build models and enable both AI research and commercialization
Neural network compatibility and integration
Choosing the best neural network framework is a challenge for data scientists. As there are many AI tools in the market, it is important to choose the best AI tool for implementing the neural network framework. However, once a model is trained in one AI tool, it is hard to integrate the model into other frameworks.
To solve this problem, tech giants like Facebook, Microsoft, and Amazon are cooperating to build Open Neural Network Exchange (ONNX) to integrate trained neural network models across multiple frameworks. In the future, ONNX is expected to become an essential technology for the industry.
Automated machine learning
AutoML supports companies to solve complicated business cases. With this technology, analysts won’t need to go through manual machine learning training processes. They can even evolve new models that can handle future AI challenges. As a result, they will focus on the main case instead of wasting time for understanding the workflow.
AutoML also offers customization for different business cases. This enables flexible models when you combine data with portability. To learn more about AutoML, you can check our article.
Advances in collaboration in AI model building
Improved tools lower the bar for model building but still human ingenuity is a key ingredient in AI models. Data science competitions help companies attract thousands of data scientists to work on their problems.
In the past, challenges like data confidentiality slowed the growth of such platforms. However, modern encryption techniques are enabling companies to share their data publicly and benefit from the wisdom of crowds without giving away confidential information.
What are the future technologies to be enabled by AI?
AI use cases will shape the development of AI. Availability of capital depends on use cases and more valuable use cases would motivate companies and government to invest more.
The improvement of AI will make our intelligent systems even more intelligent. Our cars will drive themselves, houses will adjust their electricity usage, and robots will be able to diagnose our illnesses. In other words, AI will cover more in our lives and will automate our daily tasks. Here are a few use cases of AI technologies that currently either exist in quite limited functionality or limited scope (research projects). Improvement of these technologies will unlock significant value.
AI assistants
AI-based medical diagnosis
Autonomous payments
Autonomous vehicles
Bionic organs
Conversational agents
Smart cities
Smart dust
Cloud computing based use cases
Cloud computing aims to create a system where you can achieve computing functions whenever you want. According to Gary Eastwood from IDG Contributor Network, cloud computing and AI will fuse in the future.
The integration of AI will help AI models to access information from the cloud, train themselves and applies new insights into the cloud. This enables other AI models to learn from these new insights. This fusion improves calculation power and the capability of treating many data and intelligence.
The possible use cases of cloud computing include AI-lead drones, sensor networks, and smart dust.
Extended Reality (XR)
Besides technologies like Virtual Reality or Augmented Reality, start-ups are experimenting with bringing touch, taste, and smell to enhance these immersive experiences with the support of AI technologies. While Extended Reality (XR) may bring several security issues in the future, XR will be essential to improve worker productivity and the customer experience in the future.
According to Accenture, the designers at Volkswagen can experience the car’s look, feel and drive—spatially, in 3D—thanks to XR tools.
Convergence of IoT and AI
Another trending technology, IoT, will merge with AI technologies in the future. AI can be used in IoT platforms in use cases like root cause analysis, predictive maintenance of machinery or outlier detection. Devices like cameras, microphones, and other sensors collect this data from video frames, speech synthesis, or any other media. Then, it is trained in the public cloud environment with advanced AI technologies based on neural networks.
Future of Quantum computing can be a game-changer in fields such as, cryptography, chemistry, material science, agriculture, and pharmaceuticals once the technology is more mature.
Quantum computing has a dynamic nature, acting as a useful solution for complex mathematical models, such as:
Encryption methods have been designed to take centuries to solve even for supercomputers. However, these problems could possibly be solved within minutes with quantum computing.
Even though the modeling of a molecule does not seem to happen in the near future with classical computing, quantum computing can make it possible by solving equations that impede advances in extracting an exact model of molecules. This development has the potential to transform biology, chemistry and material science.
In this article, we explain what quantum computing is, where it can be used, and what challenges might impede its implications.
What is quantum computing?
Wikipedia describes quantum computing as ” the use of quantum-mechanical phenomena such as superposition and entanglement to perform computation.”
The quantum computer concept brings a completely different perspective to the classical computer concept. Classical computers work with key-like structures that open and close, which is called bits. However, quantum computers work with interdependent and nonlinear structures called qubits. Feel free to visit our earlier article on quantum computing to learn the basic concepts for qubits and quantum computing.
Shortly, qubits have two different property that is different than the whole concept of classical computing. Entanglement is a property of qubits that allow them to be dependent of each other that a change in the state of one qubit can result and immediate change in others. more than one state during computation. Superposition states that qubits can hold both 0 and 1 state at the same time.
Why is the future of quantum computing important now?
More complex problems are arising
As technology advances, the problems encountered are getting more complex. Quantum computing offers a solution for complex problems like protein modeling. The latest global crisis caused by COVID-19 shows that scientists need a different tool to model a single protein and deactivate it. Another example of an exponential rise in complex problems can be energy usage.
As the human population increases and consumption rate increases exponentially, more complex problems like optimization of sources are arising. Quantum computers can be used to encounter the limitations of complex problems by using the physics of quantum mechanics.
Supercomputers are limited to solving linear problems
Classical computing is a convenient tool for performing sequential operations and storing information. However, it is difficult to find solutions to chaotic problems since it is modeled on the basis of linear mathematics.
Quantum computing seems to be a suitable candidate in solving nonlinear problems as it has nonlinear properties of nature. That being said, quantum computers are not suitable for all kinds of computation.
Don’t hesitate to read our state of quantum computing article, where we discuss why quantum computing is important and why tech giants invest in this technology.
What are the main trends/subjects for quantum computing?
1- Quantum Annealing
Quantum annealing is already commercially available with today’s technology by D-wave. We already discussed quantum annealing in-depth, don’t hesitate to visit.
2- Quantum Circuits
A quantum circuit consists of quantum gates, initialization & reset structures that enable quantum operations and calculations on quantum data.
A qubit can be thought of as a unit of information and the quantum circuit is the unit of computation. As quantum circuits developed to make quantum calculations become widespread, the power of quantum computing will be reflected in daily life.
3- Quantum Cloud
Cloud-based quantum computing is a method for providing quantum computing by using emulators, simulators or processors through the cloud. Quantum computing systems cover very large volume and operate temperatures at just 15 millidegrees above absolute zero.
Given the difficulty of deploying these systems, it is a necessity with today’s technology to carry out the operations desired to be performed over the cloud. Feel free to read our extended research on cloud-based quantum computing.
4- Quantum Cognition
Quantum cognition aims to model concepts such as the human brain, language, decision making, human memory, and conceptual reasoning by using quantum computing. The quantum cognition is based on various cognitive phenomena defined by the quantum theory of information in order to describe the process of decision making using of quantum probabilities.
5- Quantum Cryptography
Quantum cryptography aims to develop a secure encryption method by taking advantage of quantum mechanical properties. Quantum cryptography aims to make it impossible to decode a message using classical methods. For example, if anyone tries to copy a quantum encoded data, the quantum state is changed while trying to attempt.
6- Quantum Neural Networks(QNN)
QNNs are a combination of classical artificial neural network models with the advantages of quantum computing in order to develop efficient algorithms. QNNs are mostly theoretical proposals without full physical implementation. applications of QNN algorithms can be used in modeling networks, memory devices, and automated control systems.
7- Quantum Optics
Quantum optics is an area that examines the interaction of photons with particles and atoms. Further research on this subject provides a solution to problems encountered in semiconductor technology and communication. In this way, quantum computing can enable further development of classical computers.
What are the possible applications of quantum computing in the future?
Optimization
Many optimization problems are searching for a global minimal point solution. By using quantum annealing, the optimization problems may be solved earlier than using supercomputers.
Machine Learning / Big data
ML and deep learning researchers are seeking for efficient ways to train and test models using large data set. Quantum computing can help to make the process of training and testing faster.
Simulation
Simulation is a useful tool to anticipate possible errors and take action. Quantum computing methods can be used to simulate complex systems.
Material Science
Chemistry and material science are limited by the calculations of the complex interactions of atomic structures. Quantum solutions are promising a faster way to model these interactions.
There are numerous industry-specific applications of quantum computing in the future. For more information about quantum computing applications, please read our previous research.
What are the key challenges in the future of quantum computing?
Deciding what approach will work
There are different approaches in the implementation of quantum computing. Since quantum computerization and quantum circuits create high investment costs, trial and error of all different approaches will be costly in both time and financial terms. Different approaches for different applications seem to be the most likely solution now.
Currently, some approaches explored by QC companies are analog quantum model, universal quantum gate model and quantum annealing.
For example, Microsoft’s approach is called topological qubit method under the quantum gate model for mass production of qubits.
D-wave built the first commercial hardware solution for quantum annealing. Quantum annealing is the most likely approach to be commercialized in the near term for solving complex mathematical problems. Check out our research on how quantum annealing works and how businesses can use it.
Manufacturing stable quantum processors and error correction
In order to take advantage of the properties of quantum mechanics, it is needed to perform manipulations at smaller scales, sometimes smaller than an atom. Small scales cause stability and error verification problems.
Quantum researchers state that error-correction in qubits is more valuable than the total number of qubits obtained. Since qubits cannot be controlled with accuracy, it remains a challenge to solve complex problems.
Maintaining the extreme operating conditions
In order to increase stability and control qubits, IBM keeps temperature so cold (15 milliKelvin) that there is no ambient noise or heat to excite the superconducting qubit. Keeping the temperature so low also creates stability problems in itself. For broad commercialization of a quantum computer or processor, operating conditions should be improved.
Quantum researchers are looking for ways to use quantum processors at higher temperatures. The highest operating temperature has been reached recently. 1 Kelvin, ie -272 degrees, was recorded as the highest operating temperature. However, it seems to take more time to operate these systems at room temperature.
Problems such as stability and error correction are dependent on technology investment, research resources and developments in quantum mechanics. Different organizations are trying to obtain the most accessible quantum computer technology by trying different methods. It will take a while to see which approach will bring success in different areas.
Spare yourself the trouble and delay learning anything about quantum computing until 2022 eoy unless you are working on:
a problem that is not solvable in reasonable time with current computers (e.g. building deep artificial neural networks with millions of layers or simulating molecular interactions). Such problems are common and almost all Fortune 500 companies could benefit from quantum computers
Cryptography, or at an intelligence agency or need to transmit nation or mega corporation level secrets
quantum computing itself
If you are in one of these fields, quantum computing has the possibility to transform your field in a few years. If not, check back in 2023, technology may have progressed to the point that others may also need to learn about quantum computing.
As non-technical corporate leader, what should I do about quantum computing?
If you are working on cryptography, or at an intelligence agency or need to transmit nation or mega corporation level secrets, stop relying on cryptographic methods that rely on factoring large integers. There are already quantum-ready alternatives as we discuss in the use cases section.
If you are a problem that is not solvable in reasonable time with current computers, start exploring quantum computing. This article will explain you quantum computing and explain the ecosystem so you can find partner companies who can help you explore how quantum computing can solve your computing challenges. Do not expect immediate results though. Even though quantum computing companies cite many large companies among their customers, these tend to be PoCs with limited scope. Quantum supremacy (quantum computing being superior to classical computing) has not yet been proven for any practical application.
What is Quantum Computing?
Quantum computing involves non-classical computation which is proved to be superior to classical computation in certain important problems such as factoring large integers.
Classical computation is the foundation of almost all computing done by machines today. This includes all computing such as cloud computing, computing on your desktop, mobile or wearable device. I used the phrase “almost all” because there are also quantum computing experiments performed by researchers which should also be classified as computing. Classical computation relies on deterministic bits which have observable states of 0 or 1. As with classical (or Newtonian) physics, this is a pretty intuitive and practical approach. However, it is not efficient at modelling quantum phenomena or dealing with probabilities.
As you may remember from your high school years, Newton’s formulas are quite accurate for macro particles moving at speeds that are significantly slower than the speed of light. However, such a classical (or Newtonian) view of the world is not accurate at the molecule level or at speeds close the speed of light. This is because all matter display wave-like properties and are non-deterministic. Modeling them with bits that also display wave-like properties is more efficient.
Capability to model phenomena about molecules or particles moving close to the speed of light may seem interesting but not so useful. However, it is extremely useful:
Modelling molecule level interactions can unlock new insights in chemistry, biology and healthcare.
Quantum computing is effective at modelling probabilities and permutations as quantum mechanics is non-deterministic, certainty in classical physics is replaced with probabilities. This allows quantum computers to break RSA, possibly the most widely used cryptographic method. For example, you rely on RSA when you rely on as you transmit your credit card information to an online merchant.
For a more visual representation of how a photonic quantum computer works, you can check out this video from one of the vendors. There various approaches to building quantum computers and photonic is one approach.
Why is quantum computing relevant now?
Quantum computers are hypothesized to be superior to classical computation in certain important problems. Recent developments suggest that this could become the reality even though timelines for scientific progress are hard to estimate.
There have been significant scientific advances in the field:
Google recently claimed to prove quantum supremacy. The benchmark was simulating the outcome of a random sequence of logic gates on a quantum computer which is the task where quantum computers can possibly have the largest advantage over classical computing. While this is not a commercially useful computation, the fact that a quantum computer surpassed state of the art classical computers is still an important milestone.
In 2015, Google claimed that quantum annealers have performed orders of magnitude more efficiently in some optimization problems when compared to simulated annealers using classical computing
Quantum computing power grows exponentially with each qubit rather than linearly as in the case of linear bits due to the multi state computational capability of qubits. For example, a quantum computer with 1 qubit can simulate 2 classical bits, 2 qubits can simulate 4 classical bits, 3 bits can simulate 8 classical bits etc. This makes exponential quantum computing power growth feasible.
There is significant investment in this space:
Most mega tech companies such as Fujitsu, Google and IBM have been investing in quantum computing. In addition, startups such as D-Wave Systems raised >$200m to tackle the problem.
Number of qubits in quantum computers have been increasing dramatically from 2 qubits in 1998 to 128 qubits in 2018
How does it work?
Quantum computing allows developers to leverage laws of quantum mechanics such as quantum superposition and quantum entanglement to compute solutions to certain important problems faster than classical computers. As usual, we kept this as simple as possible, however keeping this simple was the hardest how it works section we have ever written!
Qubits, bits in quantum computers, have 2 advantages over classical bits: They can hold more than one state during computation and two qubits can be entangled (i.e. set to the same state regardless of their location).
Classical computing relies on bits for memory. Bits can either be in the 0 or 1 state. Typically, this is physically represented as voltage on physical bits.
Qubit is the name for memory in quantum computers. Just like bits in classical computing, they can be observed in two states: 0 and 1. However, they are subject to quantum mechanics and when they are not observed, they have a probability of 0 and 1. These probabilities (probability amplitudes to be precise), can be negative, positive or complex numbers and are added up “superimposed”. This is like adding waves in classical physics and allows a single qubit to be capable of holding 2 bits of information.
Other advantage of qubits is quantum entanglement which sets a group of qubits to the same state and qubits retain this equivalence until they are disentangled.
Though qubits are by nature probabilistic, they return a classical, single state when measured. Therefore in most quantum computers, a series of quantum operations are performed before the measurement. Since measurement reduces a probabilistic result to a deterministic one, numerous computations are required to understand the actual probabilistic result of the quantum computer in most cases.
Qubits can be implemented using various quantum phenomena and this is an area of active research and no mature solutions exist. Different quantum computers use different qubit implementations.
What are its potential use cases/applications?
Primary applications include optimization & research in various industries, cryptography and espionage. Feel free to read our article on quantum computing applications for more.
How will quantum computing change AI?
Quantum computing and AI are two of the most hyped tech of today. Combining them naturally raises sceptical eye brows as both fields have numerous sceptics doubting their potential. Sceptics are correct in that quantum computing is still a field of research and it is a long way from being applied to neural networks. However, in a decade, AI could run into another plateau due to insufficient computing power and quantum computing could rise to help the advance of AI.
Warning AI And Quantum Computer Just Shut Down After It Revealed In This Video
Warning AI And Quantum Computer Have you ever wondered what could happen if we bring together AI and Quantum Computers? Would this combo destroy our planet or give us a better understanding of the universe? AI has already become advanced, and scientists are tirelessly working to develop Quantum Computers, but what could happen when AI and Quantum Computers join forces? Recently the US government has pushed Google and NASA to stop their Quantum Computer development efforts. Why? Because they have noticed something terrifying.
You May Have Nothing To Hide But You Still Have Something To Fear NSA Watching You
That satellite can see us from here? It can see everything. I’m telling you. Now, the way they’re tracking everything, and finding out what’s going on and narrowing it down. The government may not be watching, but somebody out there is watching. And it all pins down to one thing. Who is watching you? This was once a question asked only by kings, presidents, and public figures trying to dodge the paparazzi and criminals trying to evade the law. The rest of us had few occasions to worry about being tracked. But today the anxious question — “who’s watching?”
Welcome To Secrets United States Surveillance Industrial Spy Complex 9/11 Politics
Central Intelligence Agency has been carrying out a mass surveillance program on American soil and in the U.S. with minimal oversight and the program’s uncovering is bad news for Big Tech according to documents declassified at the request of two U.S. senators. A Chinese Foreign Ministry spokesman appeared to claim the 9/11 attacks on America were an “inside job” by the US government in a tweet on Tuesday. Washington Examiner report that the Chinese Embassy did not respond to requests asking what the Chinese position is toward possible U.S. government involvement in the 9/11 attacks.
-
57:54
What If Everything You Were Taught Was A Lie?
11 days agoA Complete Guide To Expose Russia Gate Conspiracy Timeline Will Shock You Open Your Eyes Now
2.04K14 -
16:33
AndresRestart
14 hours agoHUGE Nintendo Game Release Date Info Just Got Out Apparently!?
24 -
16:49
Eat Sleep Cruise
7 days agoOur HONEST Celebration Key Review - Carnival's New Private Island in The Bahamas
18 -
8:41
nospeedlimitgermany
5 days ago $0.01 earnedBMW 316i E36 Compact 102 PS Top Speed Drive German Autobahn No Speed Limit POV
52 -
25:38
Clownfish TV
14 hours agoAmerican Eagle Sydney Sweeney Situation is INSANE...
9558 -
2:46
Blackstone Griddles
13 hours agoBetty's Breakfast Tacos on the Blackstone Griddle
4983 -
2:23
The Quiet Part
15 hours agoCanada is no longer requiring immigrants to speak English?!
2024 -
13:03
Dr Disrespect
3 days agoDr Disrespect’s BIGGEST Announcement Yet
106K43 -
21:08
GritsGG
13 hours agoRank 1 POV - Spectating Solos!
5.21K -
40:23
pewculture
1 day ago $3.39 earnedThis is the best western movie ever made! EP#25
15.8K5