Premium Only Content
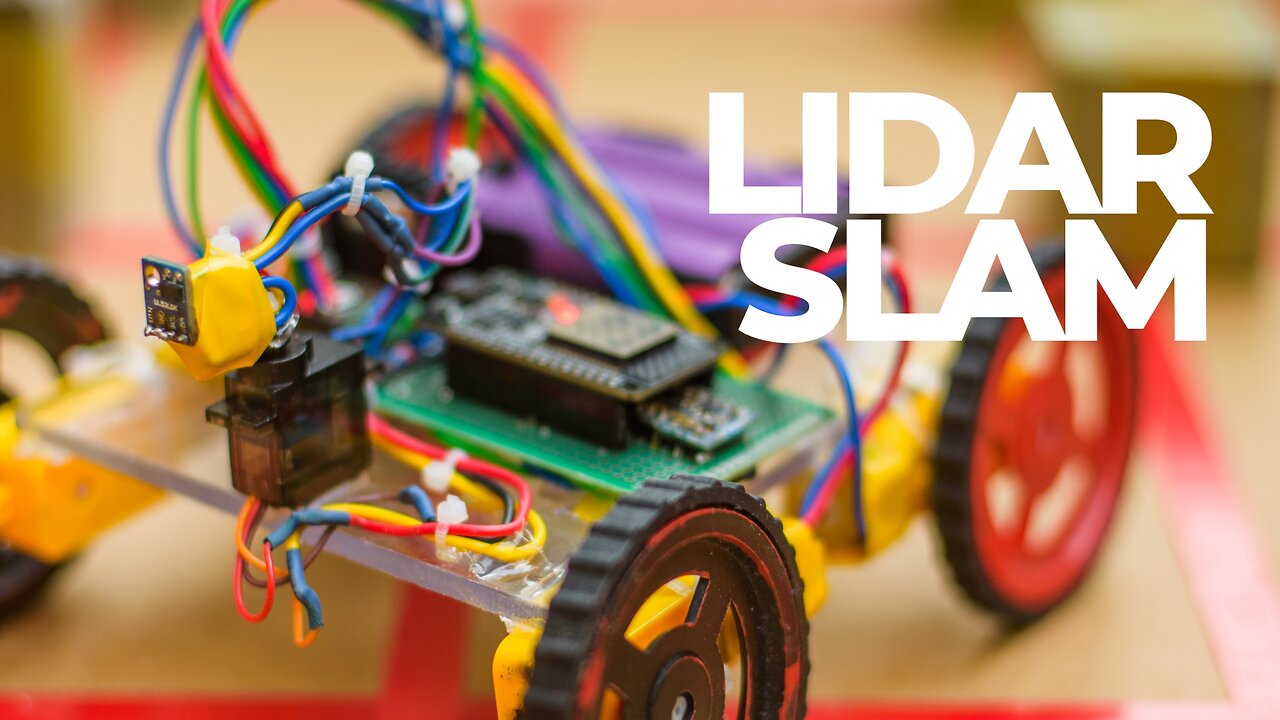
DIY LiDAR SLAM Robot from Scratch – No Libraries, No Simulations! Witness the Future of Robotics!
Embark on a groundbreaking journey into the world of SLAM (Simultaneous Localization and Mapping) as we unveil a DIY LiDAR-based SLAM system—built entirely from scratch! In this deep-dive documentary, we take you through the creation of a custom robotic car equipped with a handmade LiDAR sensor using a Time-of-Flight laser and a servo motor. From coding the entire SLAM architecture in pure Python to visualizing it with OpenCV, this project demonstrates what’s possible when you push boundaries and go beyond existing libraries.
No shortcuts. No pre-made toolkits. Just raw innovation and deep technical exploration!
We faced real-world data challenges head-on, employing particle filters, noise reduction techniques, and advanced probabilistic methods to refine our sensor data. But that’s not all—we took things to the next level by integrating AI-driven calibration to maximize accuracy and performance. This wasn't just a SLAM experiment; it was a complete robotics system, culminating in the porting of our Python project into ROS2, bringing together the power of Python and the flexibility of Robot Operating System.
In this video, you'll see the A pathfinding algorithm* in action, powered by our own custom-built library: Vagabond! 🚀 Vagabond simplifies the complexities of pathfinding, allowing users to define environments, set start and end points, and watch as the A* algorithm efficiently navigates through it all. Whether you're into robotics, AI, or game development, this library offers endless possibilities!
Highlights of the Project:
1. Handcrafted LiDAR Sensor: Created using a servo and Time-of-Flight sensor.
2. Pure Python SLAM: No pre-built libraries, everything coded from scratch.
3. Noise Reduction & AI Calibration: Smarter, more accurate SLAM data.
4. Vagabond Pathfinding: Unlock powerful algorithms like A* and Dijkstra's with ease.
5. Seamless Integration with ROS2: Bringing SLAM to life with real-time data visualization in RViz.
6. Dynamic Pathfinding & Navigation: Watch the robot adjust to real-time changes and navigate like a pro.
Ready to dive into the future of DIY Robotics? This is not your ordinary SLAM tutorial. We’re pushing the limits of what’s possible in robotics and automation—and it’s time for you to join us on this journey!
Be sure to check out and use Vagabond, our open-source pathfinding library that makes integrating algorithms like A* and Dijkstra's a breeze in your own projects:
https://github.com/Kawai-Senpai/Vagabond
#Robotics #SLAM #LiDAR #DIYRobot #AI #PythonProgramming #Pathfinding #OpenCV #ROS2 #AstarAlgorithm #Automation #TechInnovation #VagabondLibrary
-
LIVE
The Shannon Joy Show
2 hours ago🔥🔥Running Scared!! Speaker Mike Johnson SHUTS DOWN The House To Avoid The Massie Vote To Release The Epstein Files!🔥🔥
252 watching -
LIVE
Grant Stinchfield
1 hour agoIt's NOT Mortgage Fraud... It's Election Fraud! Strip Sen. Schiff of His Seat Now!
72 watching -
45:25
The Rubin Report
2 hours ago'Shark Tank' Legend Destroys Economy Narrative w/ One Fact
26.6K6 -
1:01:05
VINCE
4 hours agoOBAMA: Is It Treason Then? | Episode 91 - 07/23/25
232K116 -
1:14:59
The Officer Tatum
2 hours agoBREAKING: Tulsi Gabbard RELEASES NEW EVIDENCE On Obama, + MORE | EP 145
45.4K8 -
1:30:29
Caleb Hammer
19 hours agoSeptum Piercing Freak Makes Financial Audit History
25.1K3 -
1:28:59
MYLUNCHBREAK CHANNEL PAGE
1 day agoLand of Mu and Beyond
26.6K10 -
37:00
Rethinking the Dollar
2 hours agoObama Files EXPOSED: The Distraction You Didn’t See Coming! | Morning Check-In: Let's Talk...
19.4K4 -
1:14:10
Dear America
4 hours agoTRUMP Reveals MASSIVE Evidence Against OBAMA!! “ He’s The Ringleader” + Maxwell Subpoena APPROVED!!
92.4K151 -
2:02:47
Badlands Media
3 hours agoBadlands Daily: July 23, 2025
40.4K6