Premium Only Content
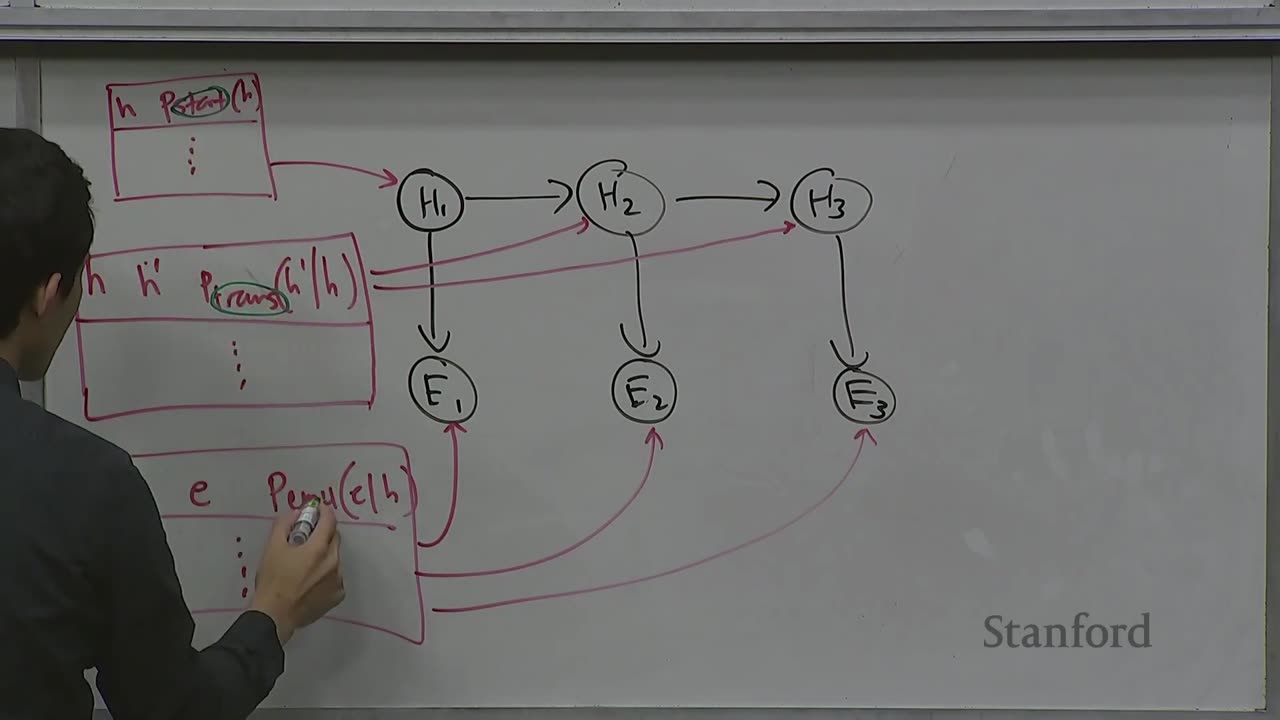
Bayesian Networks 3 - Maximum Likelihood | Stanford CS221: AI (Autumn 2019)
Topics: Bayesian Networks
Percy Liang, Associate Professor & Dorsa Sadigh, Assistant Professor - Stanford University
http://onlinehub.stanford.edu/
Associate Professor Percy Liang
Associate Professor of Computer Science and Statistics (courtesy)
https://profiles.stanford.edu/percy-l...
Assistant Professor Dorsa Sadigh
Assistant Professor in the Computer Science Department & Electrical Engineering Department
https://profiles.stanford.edu/dorsa-s...
Bayesian Networks 3 - Maximum Likelihood | Stanford CS221: AI (Autumn 2019)
Stanford Online
215
Likes
15,346
Views
2020
Dec 17
For more information about Stanford’s Artificial Intelligence professional and graduate programs, visit: https://stanford.io/2Zlc5Iu
Topics: Bayesian Networks
Percy Liang, Associate Professor & Dorsa Sadigh, Assistant Professor - Stanford University
http://onlinehub.stanford.edu/
Associate Professor Percy Liang
Associate Professor of Computer Science and Statistics (courtesy)
https://profiles.stanford.edu/percy-l...
Assistant Professor Dorsa Sadigh
Assistant Professor in the Computer Science Department & Electrical Engineering Department
https://profiles.stanford.edu/dorsa-s...
To follow along with the course schedule and syllabus, visit:
https://stanford-cs221.github.io/autu...
0:00 Introduction
0:18 Announcements
2:00 Review: Bayesian network
2:57 Review: probabilistic inference
4:13 Where do parameters come from?
4:37 Roadmap
5:02 Learning task
6:29 Example: one variable
12:06 Example: v-structure
14:48 Example: inverted-v structure
20:28 Parameter sharing
21:35 Example: Naive Bayes
26:05 Example: HMMS
33:40 General case: learning algorithm
36:14 Maximum likelihood
41:05 Scenario 2
42:45 Regularization: Laplace smoothing
44:14 Example: two variables
49:09 Motivation
49:49 Maximum marginal likelihood
52:59 Expectation Maximization (EM)
-
1:03:09
In The Litter Box w/ Jewels & Catturd
21 hours agoPardoner-in-Chief | In the Litter Box w/ Jewels & Catturd – Ep. 706 – 12/16/2024
35.6K14 -
2:15:39
Rebel News
6 hours ago $19.11 earnedRebel Roundup | Freeland and Fraser out, Fall Economic Statement delayed (again): Ezra Levant reacts
93.9K53 -
47:32
PMG
3 hours ago"Hannah Faulkner and Sam Sorbo | PARENTS GUIDE TO HOMESCHOOL"
972 -
1:59:12
Film Threat
8 hours agoVERSUS: KRAVEN THE HUNTER + LORD OF THE RINGS: THE WAR OF ROHIRRIM | Film Threat Versus
23.9K2 -
44:42
Tudor Dixon
4 hours agoBREAKING: The Truth About the Drones Invading America | The Tudor Dixon Podcast
13.1K5 -
1:47:05
The Quartering
5 hours agoHuge Drone Update, Kamala To Run Again, CNN Commits Treason & Trump Trolls On Drones
109K54 -
2:21:16
Nerdrotic
7 hours ago $7.83 earnedThe Last Jedi Still SUCKS | Superman Returns | An FNT Square Up - Nerdrotic Nooner 451
95K9 -
LIVE
StoneMountain64
6 hours agoHIGH TIER raids while on missions
101 watching -
25:58
Page Six
5 days agoLIVE: Danny & Evan talk hot reality TV takes, Bravo news & answer fan questions! | Virtual Reali-tea
50.4K2 -
1:22:35
Russell Brand
6 hours agoScience, Faith, and Totalitarianism: A Conversation with Dr. John Campbell- SF514
142K149