Premium Only Content
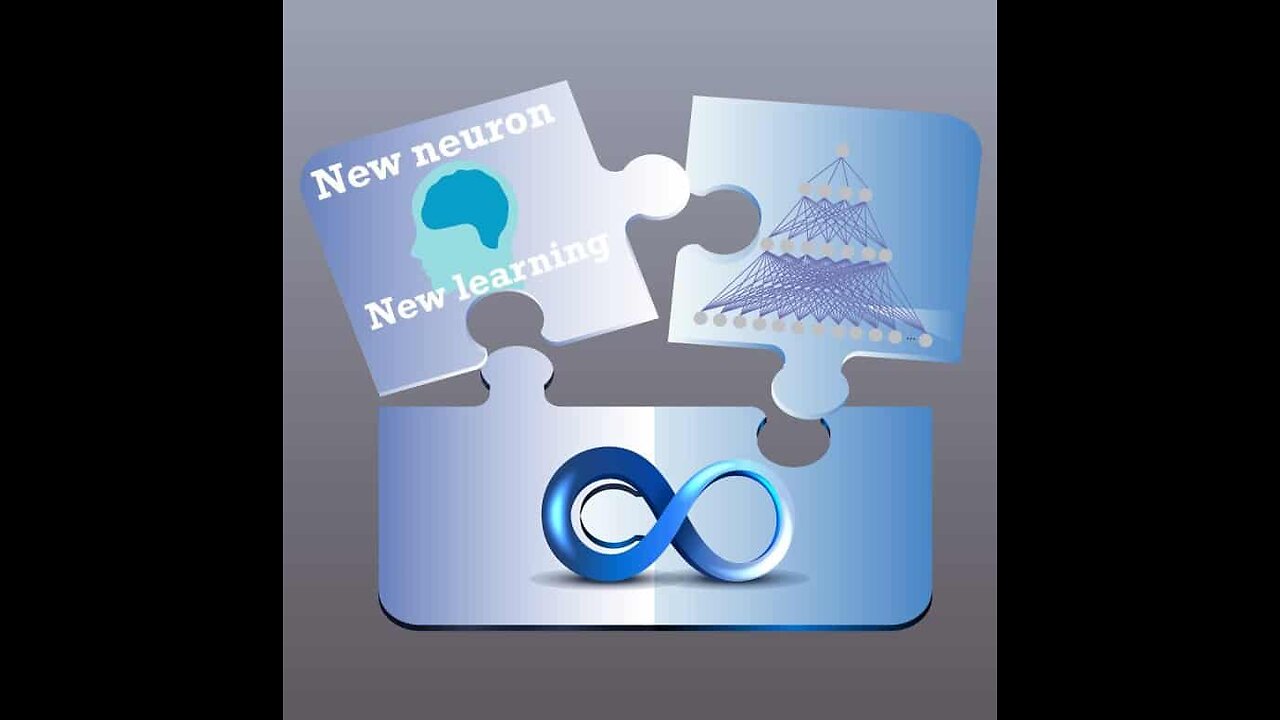
Decoding Repetitive Negative Thoughts: Machine Learning Predicts Rumination - Neuroscience News
🥇 Bonuses, Promotions, and the Best Online Casino Reviews you can trust: https://bit.ly/BigFunCasinoGame
Decoding Repetitive Negative Thoughts: Machine Learning Predicts Rumination - Neuroscience News
Summary: A team of researchers developed a predictive model to recognize patterns of persistent negative thinking, or rumination, using machine learning. Researchers hypothesized that the variance of dynamic connectivity between certain brain regions, such as the dorsal medial prefrontal cortex (dmPFC), could be associated with rumination. Brain activity was measured in participants using functional Magnetic Resonance Imaging (fMRI). This innovative model may provide a valuable biomarker for depression, aiding in early detection and monitoring treatment progress. Key Facts: The research team successfully trained machine learning models to approximate rumination scores based on participants’ fMRI data. Of all the Default Mode Network regions, only the model based on the dorsal medial prefrontal cortex (dmPFC) was successful at predicting rumination scores. The model was also successful at predicting depression scores in actual patients with Major Depressive Disorder (MDD), highlighting its potential as a valuable biomarker for depression. Source: Institute for Basic Science Our minds often get trapped in repetitive thoughts, such as past mistakes, regrets, insecurities, or unresolved conflicts. This pattern of persistent negative thinking, called rumination, can have detrimental effects on mental health, leading to conditions like depression and anxiety. Recognizing rumination as a major risk factor for depression, researchers have been working to identify its neural signature and develop early detection methods. Of all the DMN regions, only the model based on the dorsal medial prefrontal cortex (dmPFC) was successful at predicting rumination scores in healthy participants. Credit: Neuroscience News A team of scientists led by KIM Jungwoo from the Center for Neuroscience Imaging Research (CNIR) within the Institute for Basic Science (IBS), in collaboration with researchers from the University of Arizona and Dartmouth College, conducted a study to develop a predictive model of rumination by using the power of machine learning. Previous research has linked a network of brain regions called the ‘default mode network’ (DMN) to rumination. However, the specific region responsible for individual differences in rumination remained unclear. The team hypothesized that the variance of dynamic connectivity, which measures the stability of interactions between brain regions over time, could be associated with rumination due to its temporal persistency. To test this, they utilized functional Magnetic Resonance Imaging (fMRI) to measure brain activity in healthy participants at rest. Using the variance of dynamic connectivity between each DMN region and brain regions across the entire brain as inputs and self-report measures of rumination scores as outputs, the researchers trained machine learning models to approximate rumination scores based on participants’ fMRI data. Of all the DMN regions, only the model based on the dorsal medial prefrontal cortex (dmPFC) was successful at predicting rumination scores in healthy participants. Additionally, the dynamic connectivity between the dmPFC and the inferior frontal gyrus, as well as the cerebellum, was found to be particularly important in predicting rumination. These findings highlight the significance of the dmPFC in rumination and depression, which is in line with previous research linking that region with high-level, reflective processes in individuals. Notably, the model was also successful at predicting depression scores in actual patients with Major Depressive Disorder (MDD). Hence the model shows promise as a valuable biomarker for depression, aiding in the identification of individuals at risk and monitoring treatment progress. By shedding light on the neural basis of rumination and its relevance to depression, this study contributes to the advancement of mental health research and may lead to more effective interventions and improved outcomes for individuals with depression. Professor WOO Choong-Wan, the lead author, stated, “The dynamic patterns of natural thought streams greatly influence our mood and emotional states. “Rumination is one of the most important thought patterns, and this study shows that the tendency to ruminate could be decoded from brain connectivity mea...
-
57:20
State of the Second Podcast
7 days agoThe Inventor of Bump Stock Fights Back! (ft. Slide Fire)
11.5K4 -
1:04:12
PMG
1 day ago $11.69 earned"I’ll be DRONED for Christmas!"
44.9K11 -
23:38
RealitySurvival
1 day agoBest Anti-Drone Rounds For Self Defense
26.6K4 -
57:43
barstoolsports
17 hours agoBest Shot Wins The Game | Surviving Barstool S4 Ep. 7
214K10 -
1:52:24
Kim Iversen
13 hours agoLuigi Mangione Charged With TERRORISM | Liz Cheney Accused Of WITNESS TAMPERING, Faces 20 YEARS IN JAIL
119K159 -
6:50:10
Akademiks
14 hours agoJay Z says he aint NEVER been friends w/ DIDDY! Bhad Bhabie lost her man? Travis Hunter Down Bad?
121K14 -
2:27:04
AirCondaTv Gaming
12 hours ago $24.26 earnedWar Thunder - Tankering Around for That 10 Bomb
63.1K5 -
4:19:05
SpartakusLIVE
15 hours agoThe MACHINE locks in for 12-hour POWER stream
45.1K1 -
1:58:40
Robert Gouveia
14 hours agoJ6 Coverup: Prosecute LIZ CHENEY; NY Judge REJECTS Immunity; Trump Breaks Gag?
177K94 -
2:22:06
WeAreChange
13 hours agoPSYOP Spreads: Drones Shut Down Airport In New York!
115K52